Presented by:
Editor's note: This is the first of a two-part series exploring the role of Big Data in the upstream industry.
This article appears in the E&P newsletter. Subscribe to the E&P newsletter here.
Big Data isn’t new to the upstream industry. However, as the industry undergoes historic transformation toward a low-carbon future, data experts from Schlumberger, Earth Science Analytics and Peloton offered in-depth insights on how Big Data in the upstream sector has revolutionized over the past few years and what role it will play in optimizing efficiencies moving forward.
Executives in this wide-ranging discussion included:
- Jamie Cruise, Head of Products, Data, Schlumberger
- Eirik Larsen, co-founder and customer success officer, Earth Science Analytics
- Jocelyn McMinn, Product Manager, Peloton Frac
E&P: In the current environment of uncertainty as the industry recovers and transforms, how can oil and gas companies benefit from Big Data?

Schlumberger
Cruise: We are witnessing a time of fundamental change in the energy industry as the world intensifies efforts to reach net-zero emissions. At the same time, we cannot overlook that world demand for energy is continuing to rise, as more countries strive to increase their quality of healthcare, education and living standards.
At Schlumberger, our goal is to provide technology that unlocks access to energy for the benefit of all. All studies point to hydrocarbons remaining an important part of the energy mix for decades to come; however, they will be developed and managed more sustainably. Emissions of greenhouse gases in oil and gas operations will be dramatically reduced, and we will see a shift in how energy companies are focusing their investments.
Exploration has decreased significantly in the past five or so years, and research indicates exploration will enter a period of gradual decline from around 2030 while demand for hydrocarbons will decline at a considerably slower rate.
This means energy companies are looking to squeeze as much as they can from their existing assets, which translates into step-out appraisal and development from existing fields and increasing recovery rates from already producing reservoirs.
Traditional oil and gas exploration will evolve into oil and gas discovery of new or additional hydrocarbons from mature areas, and this is where Big Data will play a significant role. We can offset the exploration decline to some degree by entering a new era of processing and analytics, leveraging new data-driven decision-making technology. This means reducing the cycle time it takes to make decisions—in many cases from months to days—while massively increasing the level of confidence you have in your decisions. This is coupled with a greatly improved ability to collaborate with teams because everyone has a common, live view of reality.
In the past two years or so, the oil and gas industry has done well in understanding the Big Data technology that we need to bring to bear in the coming two or three years. The key thing now is driving adoption and crafting the digital solutions infrastructure, and this needs to be backed up by implementation projects to populate those systems with content from our legacy sources.

Earth Science Analytics
Larsen: At Earth Science Analytics, we realize that technological advancements are helping to put seismic data and knowledge at the fingertips of not only the domain experts, but the decision makers as well. With ongoing uncertainty in the market, an environment of trying to achieve more with less is prevalent.
Big Data is helping to provide one solution to market challenges, providing the ideal platform to make the most of the seismic data that operators have at their disposal, without a requirement for further geoseismic surveys or increased recruitment.
Operators now have the potential to combine seismic data with artificial intelligence [AI] technology, backed by cloud computing, supported by data analytics. By embracing Big Data, the potential is there for operators to undertake geoscience activity for exploration and production in hours as opposed to days and weeks, and with greater efficiency than ever before.
At Earth Science Analytics, our ambition has always been to provide AI solutions built around enhancing the profitability of E&P workflows and decision-making quality in the search for oil and gas. We introduced our EarthNET software to the market to provide a solution that delivers faster, cheaper and more accurate predictions when using AI for predictions of rock and fluid properties in the search for and recovery of oil and gas.
Industry challenges post-downturn will persist, but those operating in the energy sector are resilient and resolute. Positives and cause for optimism have always been a large part of our industry, and the adoption of and the current riding of the wave of ongoing digitization is one such example. As this digital transformation continues at pace, the advancement and increased solutions in a geoscience context will help support future oil and gas recovery.
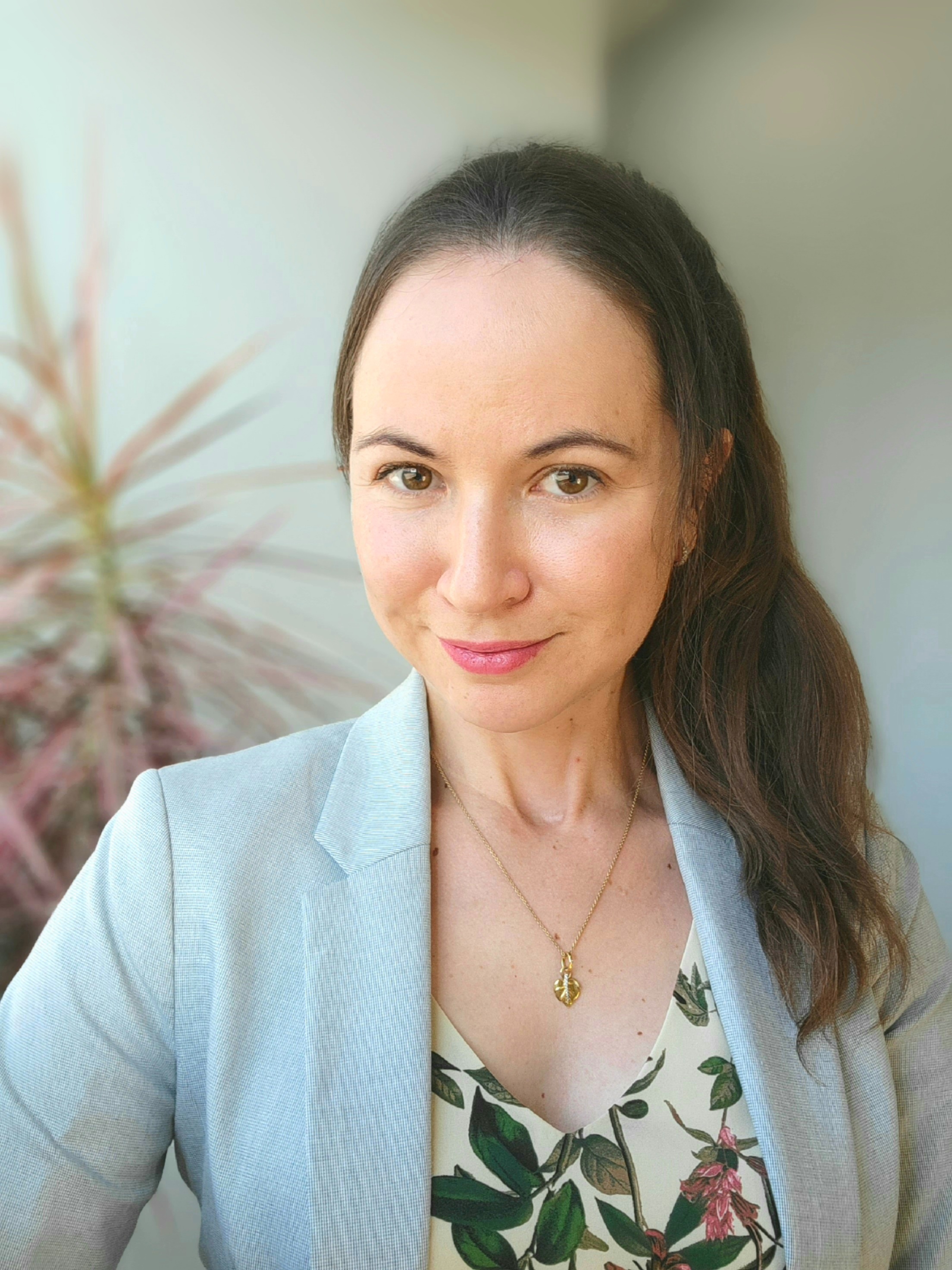
Peloton Frac
McMinn: Big data allows you to answer questions quickly and accurately, which in turn spurns
more questions, which in turn leads to more data! It’s a self-fulfilling (and rewarding)
prophecy. I like to focus on practical and immediate uses of data collection, so I can
speak to specific questions and answers I’ve seen from implementing a data
strategy. Questions such as,
- What casing string should we be running? Are there sub-areas where we can get away with a more cost-effective string?
- How can I optimize my infill drilling and fracturing schedule while minimizing risk of frac communications?
- How can I quickly assess Fracture driven interactions and develop a mitigation strategy?
- Is our operational efficiency improving and by how much?
- How much can I reduce my consumables without reducing complete effectiveness or increasing non-productive time?
- How much recycled water can I substitute before additional chemistry outweighs the benefit?
The answers to these questions have seven-figure rewards, which can be realized with one-time, six-figure spends. It may have taken an army of interns to mine, aggregate and add context to the disparate sources to answer these questions, or, perhaps the information required simply didn’t exist. Now, a completions team can do so with a few clicks. Your data strategy takes out the guesswork (and the legwork) and helps you provide concrete, analytical answers to big questions.
You may have heard the proverb “The best time to plant a tree was 20 years ago. The second-best time is now” and be worried you missed the boat during the downturn, as your human resources get constrained by a rapid upswing in activity.
However, the best part about data strategy implementation is that the tools required to do so improve rapidly. Your data strategy has never taken less cost, time, or effort to create and integrate, and you can leverage the latest technology to be successful. Instead of spending to add bodies, spend to add efficiencies. Train your existing team on how to use new tools. Your completions team (and your shareholders) will reap the benefits and allow you to answer the big questions—plus create more.
E&P: Can you give a few examples of the technologies that your company is deploying for acquiring or managing data and how it helped optimize operations?
Cruise: We have a number of major customers that we have been working with on large-scale data management and analytics for the last 25 years. But it was the development and deployment of our DELFI cognitive E&P environment that has enabled the order of magnitude changes in how customers access all types of data—including unstructured data and data that have been locked in silos for years—to derive maximum value from it.
The DELFI environment is the combination of AI and data analytics with the domain expertise of our people, enabling our customers to target profound and transformative changes in the way we work. This means going from very long, sequential and deterministic workflows to work cycles that fully capture the risks and uncertainty envelope, enabling our experts to reevaluate their options and change targets in a fraction of the time.
A recent example is our work with Woodside to develop an agile reservoir modeling solution. Their objective was reducing 18 months of field development planning to a period of just 18 days. Through the rapid and accurate data analytics capabilities of the DELFI environment, and by empowering teams from Schlumberger and Woodside to work together seamlessly in trusted collaboration, we achieved eight days.

Larsen: Earlier this summer, we published our own missed pay findings following extensive work in collaboration with the Norwegian Petroleum Directorate [NPD]. This collaborative activity used the power of Big Data within our EarthNET product’s AI system. The results outlined 3D property predictions for 545 North Sea wells within the Norwegian sector, all of which we felt could be reviewed again in-line with potential missed hydrocarbon pay opportunities.
We wanted to identify, compare and contrast hydrocarbon pay opportunities in wells that were previously marked as dry, uneconomical or simply overlooked.
For our 2021 missed pay collaboration, we used NPD’s data, collated from wells in the Norwegian Continental Shelf. This was inputted into our machine learning [ML] workflow of Earth Science Analytics’ EarthNET product. The software performed 1D and 3D property predictions at wellbore and away from the wellbore using seismic data, allowing targeting of hydrocarbon opportunities in locations close to existing fields and infrastructure.
The study resulted in a significant wealth of outputs predicting subsurface properties in an accurate and robust manner, identifying significant missed and potential hydrocarbon pay opportunities. The findings, which were driven by learning data taken from predicted well curves, were supported by real-world ‘missed pay’ examples including findings from the 2009 Grosbeak discovery. Predictions generated from the study offer opportunities for operators to extract maximum value from their existing wells and explorationists to target the best value potential hydrocarbon pay, in essence acting as a workflow accelerator.
While we focused on the Norwegian North Sea, the activity has the potential to be replicated on existing fields across the world, ensuring that previously overlooked hydrocarbons at a potential cost of millions of dollars can be recovered in an efficient way. Not only will this support operational activity and future hydrocarbon recovery, it will also support the energy transition by providing a greener, less carbon-intensive source of exploration and production.
McMinn: Peloton deploys data solutions for operators, so I can talk to some of the latest technology and how it is helping our clients optimize their operations.
Integrations between Peloton Frac and WellView on the Peloton Platform are saving clients up to 90 hours per month of manual entry time, along with providing a single source of data and reducing manual entry.
We had an operator save over $42k in friction reducer on a single well just by using historic data and setting alarms to optimize loadings.
Our clients are able catch and analyze offset hits faster with integrated data streams and smart alarms, and single timestamp data sets, resulting in reduced production losses.
Another client performed a statistical analysis of a large stage-average-pressure data set, creating a dashboard to quickly assess their area, rates, and vertical depths, allowing them to decide between a P-110/L-80 tieback. This lowered cost and drilling time in low pressure areas, while mitigating the risk of overpressures in others.
I love applying the concepts of Six Sigma, or Kaizen, to a data strategy. Measure, analyze, improve, control, define are the tenant workflows that big data can assist with. Incremental improvements at all levels, from all stakeholders can move the needle more than grand ideas, and a solid data strategy lends itself to the former.
E&P: What are the key challenges of implementing Big Data in the upstream industry?
Cruise: Our industry has a rich data landscape of existing sources from a variety of different technologies, and we need to bring that data together. So there is a mechanical issue of companies connecting their existing data—getting it out of existing systems—and transitioning it to the cloud.
Thankfully, in recent years a swathe of good technology products for achieving this have been developed, in particular, AI and machine learning agents for the OSDU Data Platform and our data integration framework, that harvests content from large-shared file systems, for example seismic files or from existing databases.
The next big challenge is how to populate the data into the cloud environment. We have devised an integrated data strategy to make sure that customer data are well-curated, passed through quality control systems and integrated. If you're bringing content together from different sources, it’s important that those sources tie up and tell one story about your asset. This involves working with customers to help them with data governance, approval and QC processes [and] to make sure that when the content is populated into their new data environment, it is coherent and evergreen.
Once you have a clean pool of data available, the next challenge is to ensure you have applications integrated with it, because, ultimately, it’s applications that enable the more efficient end-to-end workflows that customers need. We're working closely in the Schlumberger team to make sure that the application teams are all managing their data life cycle and data governance via the OSDU Data Platform.
Larsen: There are several traditional barriers to entry or challenges that need to be overcome for the full adoption of Big Data, particularly within the context of geosciences.
[For] legacy systems, one of the most glaring, particularly regarding ongoing Big Data digital transformation, relates to legacy platforms. While interest and use of the technology is there, the sector is very much operating within a transitional phase. A large part of this transition will be the transfer of legacy software from existing platforms to more cloud-based solutions.
Many E&P operators will be working within their own existing legacy software packages. This in turn leads to their own data storage being set in place, and [it] limits potential for data to be shared between the legacy systems and the new cloud solutions. A key part of harnessing the positives that Big Data can deliver relates to the sharing of information, as opposed to having a data silo. However, this is being addressed, not least of all by the OSDU data platform and other open-source data solutions.
[Regarding the] type of data available, those operating in the industry now have a wealth of data available to them, but these data are so-called feature data, not label data. For real learning to take place, data need to be annotated or labeled, helping to provide context.
In the real world, data don't come pre-labeled, but without labeling taking place, the full, successful implementation of Big Data may prove a challenge to the sector.
From E&P operators’ perspective, we know they often internally undertake labeling on a small scale, but these are often inconsistent and may even focus on a particular problem they are trying to solve that day. Current labeling can often be incredibly inconsistent, but we want to support the full adoption of Big Data by delivering large-scale labeling solutions. Our intention is to facilitate the use of data-centric AI, delivering good data training sets as opposed to just data architecture models, which are already widely in place.
We understand that the labeling of data has always been a large part of the AI process and that this process is one that is traditionally labor intensive. However, with EarthNET, we provide companies with a fully configurable platform to enable teams to interactively create, manage and improve their own ML training data as quickly as possible.
McMinn: In my experience, the biggest challenge comes from human capital. Any project needs a sponsor and a lead, and resources. The energy industry has been operating on thin margins with staff at full or over-utilization. Successful implementations rely on executive sponsors believing in a data strategy, providing strong sponsorship, including giving the team resources to fill the gaps in the day-to-day operations during the project.
Unfortunately, “side of the desk” projects are common in our industry, and while they can be successful, you can usually get there cheaper and quicker by committing human assets 100% to the endeavor. The great thing is that as technology continues to improve, implementation can move towards a “side of desk” effort. It’s now as simple as getting an involved partner in that strategy, delivering what data you have, and starting to ask the big questions.
The next step is ensuring that your entire technical team has either the capability to do basic analytics, or you have a few dedicated resources to assist others. When technical staff see the power of being able to answer questions quickly and definitively around cost, efficiency, production, etc., this spurs more questions, more dialogue, and more solutions and improvements. Data strategies fuel themselves, meaning once you start, the machine keeps moving, gaining strength, and quickly outpacing any fuel (additional investment) requirements. A recent study by Harvard business review found that the companies estimating the greatest returns last year on Big Data outspent those with much smaller ROI by a factor of more than three. Fuel the machine and reap the perpetual rewards.
E&P: Moving forward, what are some key opportunities for advancing data analytics in the upstream sector?
Cruise: Schlumberger has been providing digital data management for customers for decades, but in recent years there have been huge advances in capabilities delivered, as we have already seen, by AI enablement and migration to the cloud. The opportunity in advancing data analytics in this way is scale and cost efficiency. The cloud gives us access to almost unlimited compute and storage capacity, and migration to the cloud delivers scale and cost effectiveness by reducing the legacy infrastructure required. This is a huge advantage in the upstream sector, as using new processing technologies, the likes of AI and machine learning and other automated processing techniques, improves your decision-making and makes it vastly more efficient.
There is still scope to improve how our industry uses its data to deliver more detailed insights and thereby extract even greater value. Our approach is to work with individual customers, to look at what data resources they have available and apply a combination of techniques to improve the quality of their data, and the applications extracting the value from it. This is a core component of our INNOVATION FACTORI, where customer teams can work side by side with our experts to find the best, tailor-made solutions to enhance their workflows and operations.
Larsen: Since our foundation, the aim of Earth Science Analytics has always been to improve exploration and production success for clients. In that time, we have undertaken cross-border machine learning projects, reviewed more than 90 million depth indexes and supported clients to maximize the value of their data.
One of the key opportunities for advancing data analytics will be in making the most of existing data. As noted above, the volume of seismic data available for those in the energy sector is vast. Making the most of these data will be vital to future exploration success and reviving dormant data will be one of the cornerstones of this.
Taking advantage of computer vision technology, a field of AI centered on how computers can gain high-level understanding from digital images or videos, we are seeking to digitalize thousands of documents, making them easier to access. We, as an organization, are embracing and adopting the use of AI and ML technologies, in turn supporting the ongoing digital roll-out within the sector.
We are extracting years’ worth of well and depth illustrations, tables and charts. We are using optimal character recognition and natural language processing to extract the information from the original source into digital formats. With these data sources, we are building up an online, easily accessible database, with information in place that can be searched, found, queried and visualized.
McMinn: Reliable, affordable, global high-speed internet combined with IoT. The biggest advancement I see happening in industry is having speed connectivity to all remote locations. This will be a game changer for how the industry collects, uses, and sends feedback to instrumentation and controls.
For the last 3 decades, any industry in remote locations has not been able to fully leverage real time connectivity, still grappling with shaky connections, high latency, and expensive satellites. Each modern frac pump samples over 300 data points per second, with most of that data locked behind difficult to access PLCs. Imagine instant two way communication with every asset in your value chain, no matter its location.
Operations get safer, faster, and more efficient. Energy companies on the leading edge of leveraging these systems will reap more rewards and with bleeding edge providers expanding global, reliable internet access, there’s never been a better time to plant your data strategy.
RELATED CONTENT:
Drone Tech Roundtable: Providing a Helping Hand to E&P Operators
Spotlight: An Autonomous Directional Drilling Solution for E&P Operators
E&P Case Study: Using Edge Computing to Reduce Truck Runs by 80%
A Second Wind: Lifting Mature Oil, Gas Fields with Technology
A Data-driven Approach to Subsea Asset Integrity
Recommended Reading
M&A Values: Permian Still No. 1 as U.S. Average Falls Sharply
2024-07-10 - Gas-weighted assets’ M&A values have declined with gas futures since 2022, according to J.P. Morgan Securities analysis.
BPX Expands Its US Shale Game
2024-07-10 - BPX CEO Kyle Koontz delves into development plans in the Permian, Eagle Ford and Haynesville.
Tom Ward: Mach Looks to Other Basins as Midcon Competition Heats Up
2024-07-07 - When other E&Ps were packing up and exiting Oklahoma, Mach Natural Resources went all-in on the Midcontinent. Now Mach CEO Tom Ward says the company is exploring M&A options in other basins as competition and prices creep back up.
‘Too Many’ Players: Oilfield Services Feel Permian M&A Crunch—Dallas Fed
2024-06-27 - After a historic run of E&P consolidation, oilfield services and equipment providers in the Permian are competing to woo a dwindling number of upstream customers, according to the second-quarter Dallas Fed Energy Survey.
Gas Producers Increasingly Dominating in New Shale Top Operators List
2024-06-26 - As more private oil producers are gobbled up by giant publics, the gas players are moving up the ranks of the top operators, according to a compiled list by Enverus in an exclusive partnership with Oil and Gas Investor.