A new machine learning project analyzing 545 dry exploration wells in the Norwegian North Sea identified more than 300 potential missed pay intervals.
Last year, the Norwegian Petroleum Directorate (NPD) commissioned Earth Science Analytics (ESA) to use machine learning technology to crunch well and seismic data on the wells in a bid to gain a better understanding of the region’s petroleum system and unearth potential missed in hydrocarbon-bearing zones. The NPD made the results available in August through both npd.no and Diskos.com.
“When you gather more data, you can get more out of the data,” said Arne Jacobsen, who directed this project for the NPD. “We are promoters of data sharing.”
The project, which started as a continuation of one carried out by Oil and Gas Technology Centre (now Net Zero Technology Centre) on a majority of the wells in the North Sea, was intended to provide more insight into the remaining dry wells on the Norwegian Continental Shelf (NCS), NPD geoscientist Petter Dischington said.
“This technology has come up as a way of extracting more insights from these wells that are otherwise forgotten about.”—Petter Dischington, NPD
In 2021 when the NPD announced the project, about 1,250 wildcat and appraisal wells had been drilled in the North Sea. Of those, 796 were wildcats with around 43% of those being reported as discoveries.
“We know there’s a number of dry holes. This technology has come up as a way of extracting more insights from these wells that are otherwise forgotten about,” he said. Now, there is “dry hole analysis in the entire Norwegian North Sea.”
Jacobsen said the study focused on identifying hydrocarbon intervals that were originally overlooked.
The analysis carried out by ESA uncovered 350 intervals with hydrocarbon-bearing potential, Dischington said.
“They generated log curves showing porosity, water saturation and lithology,” he said. “We had a number of wells that had the segments, most of which quite short, some several meters thick, that could be hydrocarbon-bearing.”
Dischington said the insights garnered from the project suggest there has been overlooked pay and those zones should be looked at further.
“There is still money to be made, and machine learning can get you to that point faster.”—Daniel Stoddart, ESA
Small zones indicating the presence of hydrocarbons are valuable, “not necessarily as reserves of hydrocarbons, but for understanding the petroleum systems,” he said.
And vast amounts of data underpin that better understanding.
“We pulled all the wireline data, around 5,700 km of wireline logs, and used this data for the predictions” said Daniel Stoddart, principal data geoscientist at ESA. “Machine learning is data thirsty and ESA is no stranger to handling large amounts of data.”
He said ESA did “everything in EarthNET,” which is a cloud-native machine learning-driven software ESA developed.
The first step after centralizing the data feed was to understand what data was available and check its quality before working to gain results about porosity, water saturation and lithology.
Stoddart also said the adage “rubbish in, rubbish out is very prevalent with machine learning projects.”

ESA built a six-component workflow built on fundamental principles like using all the available data and predicting missing data, Stoddart said.
“A sonic log or part of a sonic log may be missing from the wellbore. We can predict the sonic log using all the logs in the project to get a good prediction and infill where the sonic log is absent or missing a section,” he said.
“When you gather more data, you can get more out of the data.”—Arne Jacobsen, NPD
Alienor Labes, a geodata scientist at ESA who worked on the project, said the ability for machine learning to predict attributes such as missing data and then use that synthetic data is “very interesting” for geoscientists.
“Geoscientists know there is no absolute truth and that is true with machine learning as well. But the better data quality, the better the predictions will be,” she said. “With machine learning, you can also find and identify esoteric relationships between different types of data that you might have missed or not seen.”
ESA built a model and validated and benchmarked the model and the predictions both statistically but also geologically, Stoddart said.
He said the end game was “predicting pay intervals in dry wells and outside the main producing intervals, using porosity and water saturations. These parameters are interesting to the geologist when doing evaluation for prospectivity in the area.”
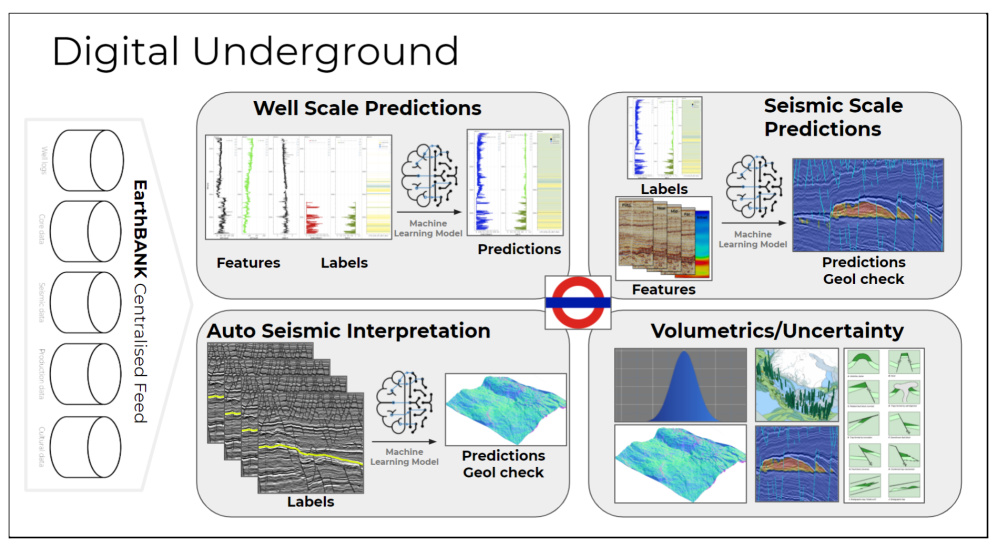
But, as Stoddart said, the term missed pay could be ambiguous.
“What’s missed pay to me is maybe not missed to you,” he said.
Of the more than 300 instances the results predicted as potentially missed pay, “we are not saying every one of those pay intervals are hydrocarbon-bearing,” he said. They are, he said, candidates for geoscientists to further explore.
He called the work a prospect portfolio accelerator.
“It’s not a silver bullet. It’s a fast way of getting to pay,” he said. The workflow “identifies the pay zone, but it’s the geoscientist who must place the data into the geological context and decide whether it makes geological sense.”
According to ESA, the approach saved a lot of time, and if the analysis were conducted again by EarthNET, it would take around three to four months, while conventional methods would take between one and two years.
“Geoscientists know there is no absolute truth and that is true with machine learning as well. But the better data quality, the better the predictions will be.”—Alienor Labes, ESA
That accelerated workflow is increasingly important, Stoddart said, as companies downsize personnel but still have the same level of work that needs to be done.
“There is still money to be made, and machine learning can get you to that point faster,” he said.
Jacobsen said he sees many further possibilities when it comes to using machine learning to process and analyze well data. A project covering producing wells “could be interesting,” he said.
And Dischington wants to see analyses done on dry exploration wells in the Norwegian and Barents Seas.
But in the meantime, he’s “really intrigued to see what the industry will do with this dataset.”
Recommended Reading
Trump Stirs Confusion with Support, Spurning of Canadian Oil
2025-01-24 - President Trump signed an order that could boost Canadian crude imports — and then said the U.S. doesn’t need Canadian crude.
Adkins: Saudi Cuts to Stay, EVs are Overrated and China Matters Less
2024-12-03 - Marshall Adkins, head of energy at Raymond James, isn’t buying the prevailing wisdom that weakening Chinese oil demand, EV encroachment and a potential OPEC supply increase are legitimate threats to the oil market.
NatGas Prices, E&Ps Take a Hit from DeepSeeking Missile
2025-01-28 - E&Ps such as Expand Energy and EQT Corp. saw share prices drop on news of less power-intensive AI, but analysts predict the natural gas market will rebound as LNG exports and overall power demand continues to increase.
NatGas Shouldering Powergen Burden, but Midstream Lags, Execs Warn
2025-02-14 - Expand Energy COO Josh Viets said society wants the reliability of natural gas, but Liberty Energy CEO Ron Gusek said midstream projects need to catch up to meet demand during a discussion at NAPE.
Comments
Add new comment
This conversation is moderated according to Hart Energy community rules. Please read the rules before joining the discussion. If you’re experiencing any technical problems, please contact our customer care team.