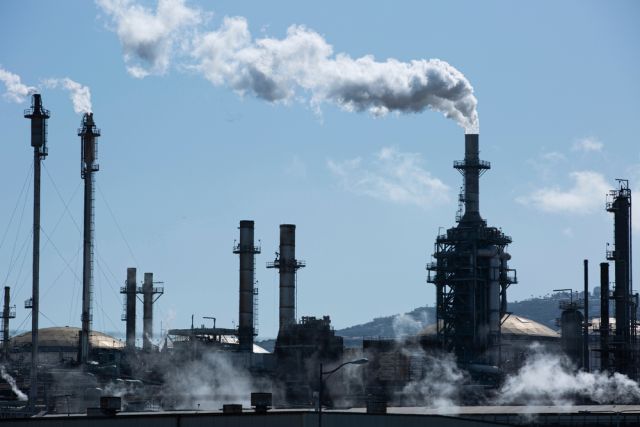
Alongside being imperceptible to the eye, gases are being emitted from hundreds of thousands of equipment pieces at sites. (Source: Shutterstock)
Perhaps it’s only inevitable that combating the widespread chaos of emissions leaks requires the talents of a mind that doesn’t work like a human’s.
“Emissions work is hard,” Raoul LeBlanc, vice president of research and analysis at S&P Global Commodity Insights, said during a recent AWS Energy Symposium.
“You’re trying to measure something that’s invisible and sometimes comes out on purpose and other times comes out on accident.”
Alongside being imperceptible to the eye, gases are being emitted from hundreds of thousands of equipment pieces at sites—each piece being unique—causing operators to deal with an enormous amount of heterogeneity. LeBlanc said operators in the energy space are not utilizing proper tools when attempting to measure and track emissions.
“We’re using a flathead screwdriver on a Phillips screw,” LeBlanc said. “Most of the data systems and the IT infrastructure that was set up was done to do oil and gas measurements and all of a sudden they’re being repurposed.”
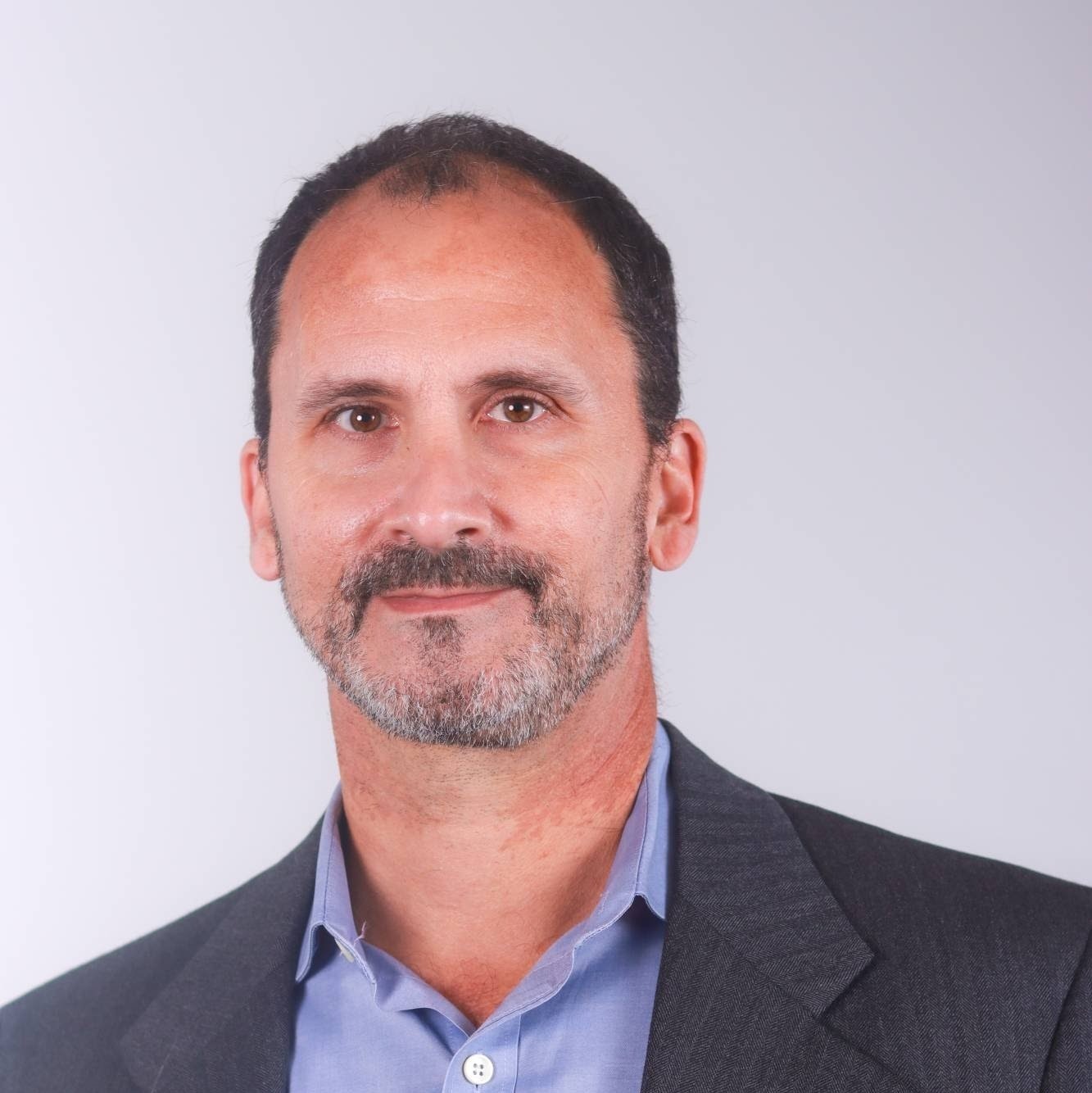
S&P believes the have a multipurpose tool that can help. Call it an artificial intelligence and machine-learning screwdriver.
But challenges remain and the scale of the problem is daunting.
The repurposing of tools originally used for standard oil and gas operations has caused issues for many operators. It can be difficult to gather, measure or interpret information without using the proper tools. The collected data either won’t be as precise or not acquired in the most efficient manner.
Another problem is the constantly changing regulatory standards and dynamics within t industry that turned the sector into the “Wild, Wild West,” he said. With the continually changing parameters preventing a standard way to collect emissions data, companies experience large amounts of “data headaches.”
These “data headaches” are further exacerbated by the dearth of new ideas when it comes to emissions management.
“You’re trying to lower emissions, and the problem is you started with the right stuff, which is the no-brainer [solutions], but now you’re starting to run out of ideas,” LeBlanc said. “All the low hanging fruit are starting to get picked and now you’re going to have to start spending real dollars.”
And while nearly every company is hard at work, inventing new data models and creating new algorithms, they oftentimes end up with the exact same solutions. S&P is working to create a solution that remedies all.
“We’re trying to create an environment in which companies can put in their proprietary confidential data and trust that we’re going to anonymize it to an degree that it can drive real models that really work to give them insight so that everybody doesn’t have to do this for themselves,” LeBlanc said.
But LeBlanc admits that there has been “no easy button” when it comes to adoption as intracompany disconnect has popped up amongst those looking to use S&P’s solution.
“You go to the people at the working level, and they’re mostly like, we are never giving away this information,” he said. “And then you go up and you start talking to the C-suite and they say, ‘You know what? We’re willing to do it.’”
LeBlanc hopes companies begin to see eye to eye as emissions is the one place where collaboration is useful, he said.
“This is a deal where you could look around at other companies and see what they have done in a similar type of asset, and apply that,” LeBlanc said. “And then we do some digital twins where I predict what the emissions for a site ought to be, and then I look at what they actually are and bam, I have outliers. That’s where I want to spend my money. That’s where I want to start my work.”
Even with the benefits of sharing information in emissions management, operators are still lacking in preparation.
“The real reason they’re unwilling to put in their data most of the time is that they have initiative overload, and they are always busy and understaffed trying to get the data together. So they tell us, our data’s not ready for this. We would love to participate in this, but our data’s not ready and it’s difficult,” LeBlanc said.
S&P’s machine learning capabilities can help operators understand their emissions by truly “lifting the hood and looking at the engine,” helping them quantify why their assets emit the way they do.
Machine learning can also enhance an operator’s predictive capabilities, allowing them to create predictive models with 10 time the data. S&P’s solution will also help with optimization as the machine would be able to search its database, find similar assets throughout the world and study what made those assets successful.
“If you can reduce your per ton cost of carbon abatement from $80 to $70 or $65, it is an enormous amount of money over the long term,” he said. “We had one company said, yeah, our plan is to spend $2 billion by 2030 to hit our goal. And imagine if you can save five, 10% of that. It starts to be quite real in terms of the money.”
LeBlanc wants to turn emissions reporting from a cost center to a value center for operators, by taking the exhaust of their monitored and measured data and allowing operators worldwide to analyze it and capitalize from it.
“As you run out of that low hanging fruit, you’re going to have to try a bunch of new things for the first time. Your company is going to have to do some things for the first time,” LeBlanc said. “Wouldn’t that be amazing if you could figure out what happened to the other people and you’re not flying blind?”
Recommended Reading
NatGas Prices Jump on Forecast of Widespread Freeze
2024-12-30 - The National Weather Service predicts a mid-month, lingering cold snap for much of the U.S., prompting natural gas prices to increase by roughly $0.50 per MMBtu.
US NatGas Prices Hit 25-Year Low at Henry Hub, Negative Territory at Waha
2024-11-11 - U.S. spot natural gas prices fell on Nov. 11 to a 25-year low at the Henry Hub. At the Waha Hub in West Texas, prices fell into negative territory.
NatGas Rally Reaches 2024 Peak, Highest Price in Two Years
2024-12-19 - Analysts say the gas market remains volatile as prices surpass $3.50/MMbtu at the Henry Hub for the first time in about two years.
NatGas in Storage Shows YOY Drop for First Time Since 2022
2025-01-03 - Despite a drop in gas in storage, prices took a hit from forecasts of warmer-than-expected weather.
US NatGas Prices Hit 23-Month High on Increased LNG Feedgas, Heating Demand
2024-12-24 - U.S. natural gas futures hit a 23-month high on Dec. 24 in thin pre-holiday trading.
Comments
Add new comment
This conversation is moderated according to Hart Energy community rules. Please read the rules before joining the discussion. If you’re experiencing any technical problems, please contact our customer care team.