
The inherent complexity of subsurface data and the need to make swift decisions demands a tailored approach. (Source: Shutterstock.com)
While the oil and gas industry is years into its digital transformation journey, there is still significant room for progress, especially in areas like subsurface operations.
Momentum for subsurface transformation has been building for years on the back of hyper-scaled cloud technology, progress toward data platforms, open industry collaboration consortiums, AI and increased vendor innovation. However, challenges such as talent and skills, trust in AI, legacy infrastructure and data systems, and proprietary data restrictions have slowed progress.
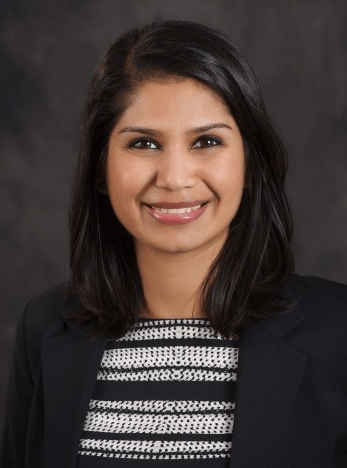
The complexity of subsurface data, coupled with the need for swift decision-making in asset development and field management, demands a tailored approach to data and AI strategies. Companies are learning that the methods that work for siloed, simpler cases don’t translate to the nuanced and data-intensive world of integrated subsurface workflows. Successful execution requires adapting the approach to unique volume, velocity and variety constraints that subsurface data imposes.
To learn how oil and gas companies can jump the transformation curve in their subsurface operations, it’s essential to first review some of the current high-value areas of opportunity around subsurface innovation. Many of these can be split into two themes: (1) prospect identification and asset characterization; and (2) drilling, completions and
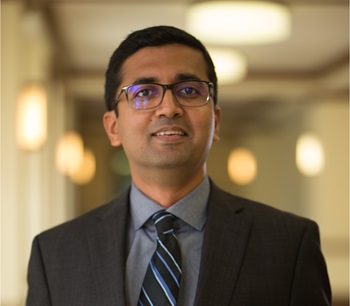
production efficiency management.
Prospect identification, asset characterization
- Seismic data processing and interpretation: AI algorithms, particularly deep-learning models, are revolutionizing 3D/4D seismic data analysis by automating the identification of subsurface structures and stratigraphic features. These models can process large seismic data sets, enhancing signal-to-noise ratio (SNR) and identifying geological features and direct hydrocarbon indicators (DHIs) with improved accuracy and speed.
- Well log and core data analysis: AI is being applied to interpret well log and core data. Sophisticated algorithms can identify lithology and rock facies, detect hydrocarbon-bearing zones, and estimate reserves more efficiently than traditional methods.
- Subsurface imaging and mapping: Advanced AI techniques are enhancing the resolution of subsurface imaging, allowing more detailed and accurate geological maps that are crucial for identifying potential drilling targets and understanding the geology of unexplored areas.
- Reservoir characterization: Machine learning (ML) techniques are used to synthesize diverse data types, including seismic attributes, well logs and production data, to create high-fidelity models of reservoir properties. These models provide insights into the spatial distribution of critical reservoir characteristics, such as porosity, permeability and fluid saturation, which are essential for accurate resource estimation and recovery planning.
D&C, production efficiency management
- Drilling and completions (D&C) optimization: AI optimizes D&C operations by analyzing real-time data from the drill bit and adjusting operational parameters. This includes enhancing rate of penetration (ROP), minimizing bit wear and preventing hazardous situations such as stuck pipe incidents.
- Well path design: AI is improving drilling of horizontal wells through efficient well path design that manages costs and avoids collision while maximizing hydrocarbon recovery.
- Production forecasting: Leveraging historical production data and real-time sensor inputs, AI models can forecast future production with more accuracy. This allows operators to make informed decisions about well interventions, facility planning and overall field management.
- Enhanced oil recovery (EOR) scenario analysis: AI is playing a key role in designing EOR strategies by simulating various injection scenarios. By predicting their outcomes, AI helps in selecting the most effective technique to increase the recovery factor of mature fields.
- Predictive maintenance of subsurface equipment: AI utilizes real-time data from sensors and historical maintenance records to predict equipment failures before they occur. This approach can significantly reduce unplanned downtime and extend the lifespan of critical infrastructure.
- Real-time reservoir management: AI enables dynamic reservoir management by continuously analyzing data from a variety of sources, including downhole sensors and production systems. This allows for the adjustment of production strategies in real time to respond to changing reservoir conditions, improve hydrocarbon recovery and manage resources more sustainably.
- Risk assessment and uncertainty reduction: AI models are critical for assessing risks associated with D&C and production activities. By analyzing geological data, operational parameters and economic factors, AI can help quantify the uncertainties and provide probabilistic outcomes, thus supporting better-informed decision-making and risk mitigation strategies.
Jumping the transformation curve
While clearly there is opportunity in subsurface operations, progress in this function has lagged. So, where should companies focus to transform?
First, leaders must leverage investment that is already in place and bring forward subsurface use cases that can be piloted and scaled. It is important that leaders and teams select use cases that can be scaled to both build trust in AI-based outcomes and to deliver business value at pace.
Second, building AI tools is not enough. Ongoing maintenance of AI systems and curating the data they consume are critical. Companies must manage AI adoption and its integration into subsurface workflows and key stage-gated decision-making.
Third, fostering a culture that embraces AI begins with educating employees on its advantages and how it can augment their roles, leading to a more rapid adoption.
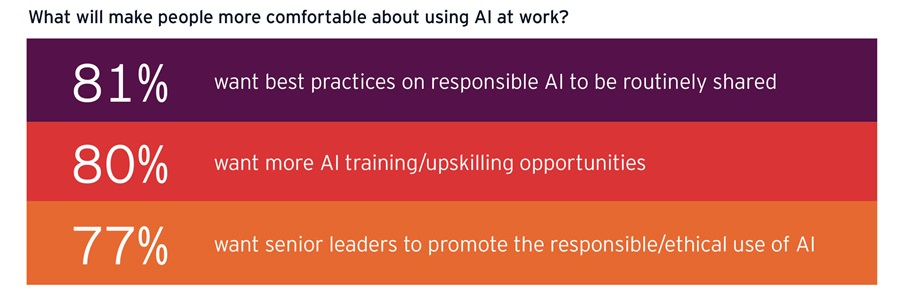
Finally, companies should focus both on establishing a streamlined decision-centric approach to data and on acquiring and integrating AI solutions in the market that have been validated by the industry. By reserving internal development mainly for proprietary technologies that provide a distinct competitive edge, companies can free up much needed resources and progress at scale.
Swapnil Bhadauria is a senior manager at Ernst & Young and serves as the EY Americas Oil & Gas Digital Operations Leader. Abhilash Krishna is a manager at Ernst & Young LLP in the consulting practice and focuses on business transformation in the oil and gas sector.
Recommended Reading
Barrio Energy Acquires 12-MW Data Center in Texas
2024-08-22 - Barrio Energy’s new 12-megawatt data center in Tyler, Texas, brings the company’s number of data center properties in the state to five.
Geospace Technologies Sells Seismic Data Nodes to SAExploration
2024-08-28 - SAExploration exercised its purchase option from an existing rental contract to make the purchase of seabed ocean bottom wireless seismic data acquisition nodes.
APA, Palantir Expand Partnership to Deploy New AI Across E&P Operations
2024-09-25 - APA Corp. will introduce new AI capabilities to its oil and gas operations with Palantir Technologies’ Artificial Intelligence Platform (AIP) software.
ZEMA Global to Acquire Morningstar’s Commodity Data Business
2024-09-23 - ZEMA Global Data Corp. is acquiring Morningstar Commodity Data to meet demand for data and analytics prompted by the global energy transition.
TGS Releases Illinois Basin Carbon Storage Assessment
2024-09-03 - TGS’ assessment is intended to help energy companies and environmental stakeholders make informed, data-driven decisions for carbon storage projects.
Comments
Add new comment
This conversation is moderated according to Hart Energy community rules. Please read the rules before joining the discussion. If you’re experiencing any technical problems, please contact our customer care team.