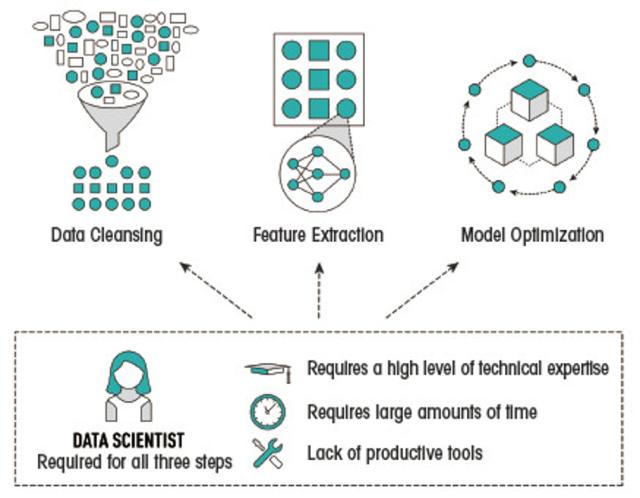
Data scientists must be skilled and require extensive lengths of time to analyze the data to determine when maintenance is needed. (Source: SparkCognition)
In an industry like oil and gas, unexpected failures can be catastrophic for many reasons. The cost of maintenance or new parts is often exorbitant, and with how much capital is committed to wells by a company per day, the lost opportunity cost from unplanned downtime can be shocking.
Despite this, the industry has never had particularly great methods of predicting and preventing unexpected failures before they can cause problems. Too many operators still take either a reactive or scheduled approach to maintenance, both of which have tremendous downsides for reliable production. Reactive maintenance runs the risk of creating safety hazards or large operational damage, while scheduled maintenance often halts operations unnecessarily. Enter predictive maintenance, which shows great promise as a new paradigm for monitoring operations but has been a challenge to implement efficiently and effectively. New technology is needed to enable oil and gas operators to truly enjoy the benefits of predictive maintenance and keep their rigs running safely and continuously.
That new technology is none other than automated model building (AMB), which aims to deliver artificial intelligence (AI) to the fingertips of industrial companies, hence ensuring the success of predictive maintenance programs without the need for large data science organizations. As an AI company working in the oil and gas space, SparkCognition already has helped oil and gas operators apply AMB to their operations with great success.
Current state
Predictive maintenance, which involves analyzing patterns in sensor data to forecast impending asset failures before they occur, is an invaluable tool for oil and gas operators. It allows companies to optimize their maintenance strategies, performing the needed maintenance on the asset while it is not in use or bringing in a replacement beforehand if the failure cannot be averted. This greatly reduces unscheduled downtime while eliminating many of the uncertainties from the drilling process.
According to research by the Electric Power Research Institute, the annual cost of scheduled maintenance is $24/ hp. Reactive maintenance costs $17/hp per year—before considering the additional costs of the safety hazards or operational damage that may be incurred by asset failure. Predictive maintenance was found to be by far the most cost-effective method, costing only $9/hp annually and including no hidden costs or dangers.
The quantitative benefits of predictive analytics also have been well documented within the oil and gas sector. McKinsey & Co. found that advanced analytics can yield as much as 30 to 50 times the initial investment within the first few months of implementation.
In spite of this, the oil and gas industry has been slow to adopt predictive maintenance, as it comes with some barriers to entry. Predictive maintenance requires data analytics, but data science talent can be scarce. Even for operations that do have an in-house data science team, the exorbitant amount of time predictive maintenance requires from it in collecting and analyzing new sensor data is neither feasible nor scalable.
Predictive maintenance also relies on the use of models based on prior data to predict asset health and operating states. Traditionally, these models were built on predefined rules worked out by human analysts. Such models can take enormous amounts of time and human talent to build, perform poorly in extreme or unusual conditions and are subject to human bias. Additionally, they are inflexible; if a single variable of the asset changes, the model is rendered useless.
AMB is the answer
AMB opens up the full potential of predictive maintenance to the oil and gas industry. AMB platforms ingest operational, sensor and historical data, including production data (oil, gas, water, etc.), sensors from compressors, pumps and vapor recovery units. Then they automatically build machine learning models that predict the operating state and remaining useful life of a given asset. This reduces the time required for the process of model creation by orders of magnitude, bringing it down to weeks or even days.
AMB also amplifies a data scientist’s work by enabling more effective model building. It gives data scientists the ability to iterate through thousands upon thousands of different models and architectures rapidly. In doing so, AMB allows data scientists and nondata scientist model builders alike to focus on their data problems rather than parameter tuning within models.
As AMB platforms leverage machine learning, models produced by AMB are continuously learning and refining themselves, allowing them to adapt to changing conditions in assets and catch the never-before- seen failures or edge cases that traditional models struggle with.
Case study
SparkCognition proved out the utility of AMB in predictive maintenance for oil and gas for a major E&P operator looking to leverage the power of AI to predict workover needs and production in oil fields.
As oil and gas maintenance operations go, workovers do not come cheap. They require large crews, heavy equipment and the complete shutdown of production. For a fairly normal well that produces an average of 50 bbl/d priced at $60/bbl, ceasing production translates to an average of $3,000 lost income per well for each day it’s not running. Add to that the costs of a workover rig with support equipment and crew at about $10,000 per day and it’s a hefty price tag. All told, workover operations can cost roughly $70,000 to $100,000 for a single well.
Unfortunately, workovers are a necessary evil of oil and gas production. On average, 10% of wells in a field require a full workover each year. Savvy operators are always on the lookout for ways to reduce this number while maximizing returns, and this operator decided to turn to AMB as a possible solution. Specifically, the operator wanted an automated, reliable solution to monitor the state of its wells, assist process engineers in identifying which wells needed maintenance (and what kind of maintenance), and predictive maintenance needs enough in advance to allow the operator to minimize downtime. It also wanted a solution that would help process engineers prioritize maintenance operations by assessing the potential return of a given well after a workover event.
Working together with SparkCognition’s AMB Darwin platform, the operator rapidly created cognitive models to predict well maintenance needs and future well production accurately. These models were created using a limited dataset with only one input per month. This set included data on well static properties, including location, reservoir characteristics, and monthly oil, water and gas production. Darwin ingested these data, extracted the 40 most relevant features and built optimized models to identify seven types of maintenance events for wells in the field.
The models Darwin built predicted workover, rod change and cleaning operation needs in 12 out of 17 wells in the field with accuracy between 70% and 80%. These predictions were made three to six months in advance, allowing the operator to plan maintenance operations. In doing so, the operator was able to improve safety and reduce its operating costs by replacing expensive emergency repair with informed, as-needed scheduled maintenance events.
The models also provided valuable insights for process engineers on well production potential. The engineers were able to maximize their returns by focusing efforts on the most promising wells.
Recommended Reading
Halliburton, Sekal Partner on World’s First Automated On-Bottom Drilling System
2025-02-26 - Halliburton Co. and Sekal AS delivered the well for Equinor on the Norwegian Continental Shelf.
Diamondback in Talks to Build Permian NatGas Power for Data Centers
2025-02-26 - With ample gas production and surface acreage, Diamondback Energy is working to lure power producers and data center builders into the Permian Basin.
Iveda Deploys New Liquid Storage Management Solution for Gulf Western Oil
2025-02-26 - IoT servicer Iveda’s LevelNOW system uses sensors to deliver real-time information about tank levels.
Innovex, SLB OneSubsea Reach Supply Agreement for Wellhead Systems
2025-02-24 - SLB Onesubsea will supply Innovex subsea wellhead systems, building on the companies’ long-standing partnership.
Analyst: Microsoft May Be Mitigating Oversupply by Ending Data Center Leases
2025-02-24 - Microsoft, the tech giant and primary benefactor of OpenAI, refuted oversupply claims to say its strategy remains the same.
Comments
Add new comment
This conversation is moderated according to Hart Energy community rules. Please read the rules before joining the discussion. If you’re experiencing any technical problems, please contact our customer care team.