From the subsurface to the production field, the conversation in upstream oil and gas has been dominated by digitalization and the potential for technologies like artificial intelligence (AI) and machine learning (ML) to deliver greater efficiency, consistency and performance improvement through automation.
But talking about the promise of technology is a far cry from achieving it.
Even though advanced solutions have the potential to transform the industry, implementation has not been rapid or uniform. Some companies are just beginning to experiment with these technologies, while others are incorporating them in day-to-day operations.
One reason for the inconsistency in adoption is that integrating advanced technologies requires a shift in thinking from mechanical to digital terms. And changing a mindset is challenging.
In some ways, this is the same sort of adjustment that took place in the 1990s when the industry began gathering sensor data from equipment for vibration analysis and condition monitoring. Analyzing performance data provided the insight required to move from planned maintenance to an early form of predictive maintenance. The value of being able to predict and plan rather than react produced tangible results. Now, there is broad acceptance that using performance data for more informed decision-making delivers efficiencies that cannot be achieved any other way.
Understanding the lexicon
Advanced technologies hold immense promise, but to extract value from these solutions, it is critical to understand how they work.
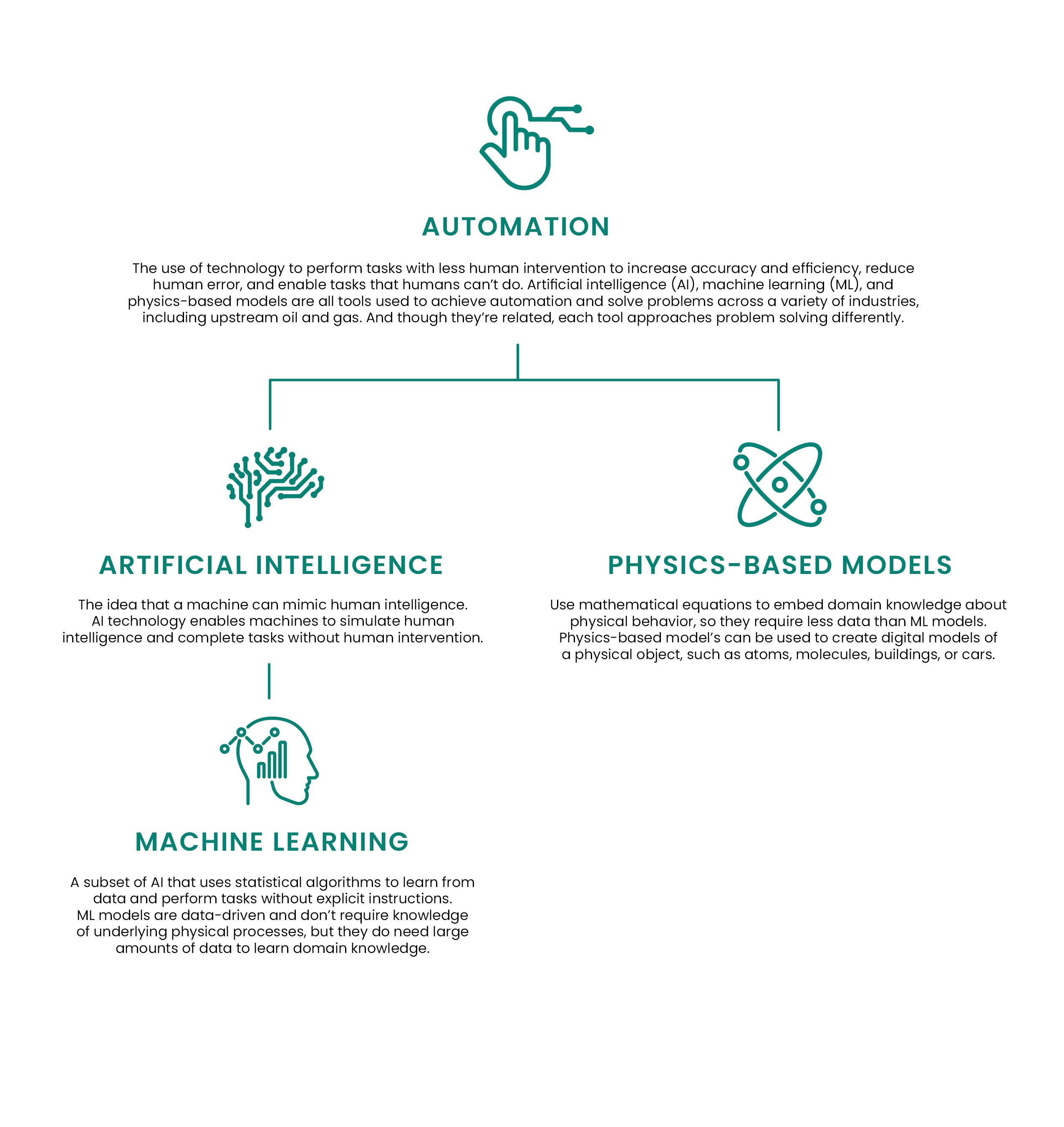
According to the results of a recent industry survey, there is confusion about what “digitalization” actually means. The fact that there are multiple definitions for digitalization makes it difficult to have a productive conversation about how it can be applied to deliver value. Agreeing on—and understanding—the terminology is a prerequisite for broadly applying digital solutions and measuring their value in terms of operational improvement.
A nearly ubiquitous digital technology is AI. AI systems are designed to perform tasks that historically have relied on human intelligence, such as understanding natural language, recognizing patterns and making decisions. AI systems can learn, reason to some degree and, in conjunction with a control system, act autonomously. Such AI systems are used in oil and gas operations to improve well planning, detect hazards like high levels of gas, identify equipment anomalies and optimize drilling operations
ML is a subset of AI in which data-driven models use statistical algorithms to learn and perform tasks without explicit instructions. This technology is used broadly in oil and gas operations. ML models examine data to recognize patterns that can be used, for example, for process optimization or identifying equipment wear and predicting potential failure conditions.
Although ML models are similar to physics-based models in that they use historical and real-time data, physics-based models function differently. They use underlying knowledge to create digital models of physical objects from small hydrocarbon molecules to multiphase flow, to equipment aging and degradation in harsh environments, to large, complex subsea installations.
Physics-based models are critical components for creating digital twins, which replicate a physical object, process or system. Constructed using all the data available, a digital twin combines real-time data, simulation capabilities and predictive analytics to transform data into actionable insights that can be applied to operations. Because a digital twin uses both historical and operational data, it is a valuable tool in the design and planning stages of a project as well as during operations.
Collectively, these technologies are enablers for automation, which allows tasks to be carried out with less human intervention or with no human intervention at all.
Drivers for automation
There are many reasons for automating operations, but they all boil down to one thing—value.
For decades, the oil and gas industry has struggled to contend with a shortage of skilled workers and increasingly relies on technology to help fill in the gaps as experienced, older workers retire. Automating processes allows novice crews to work at a much higher skill level, carrying out routine functions and delivering consistent results. For veteran oilfield workers, automating these functions streamlines operations, which allows them to avoid repetitive tasks and focus instead on higher-value or more complex aspects of their jobs.
Automation also makes it possible to deploy smaller onsite crews, which has a number of operational benefits.
Smaller crews mean fewer trips are required to get workers to and from the field, and fewer vehicles generate less emissions. This creates a pathway for reducing CO2e from well construction operations and precipitates a reduction in CO2e from every barrel of oil produced by the asset. And when automated functions are electrified, there is an even greater reduction in the environmental impact of day-to-day operations.
Automation is also revolutionizing safety, which is directly related to productivity in oil and gas operations. Automation is a way to prioritize safety by reducing the number of people required on site and consequently reducing the opportunities for injuries, incidents and accidents.
The biggest value driver, however, is that automation increases productivity. Once a process is automated, it can be optimized, which allows more value to be extracted from existing assets.
Today, automation—often embedding technologies like AI, ML and physics-based models—is changing the face of oil and gas operations, delivering on promises to drill faster and farther with better accuracy and ultimately, produce more hydrocarbons at a lower cost.
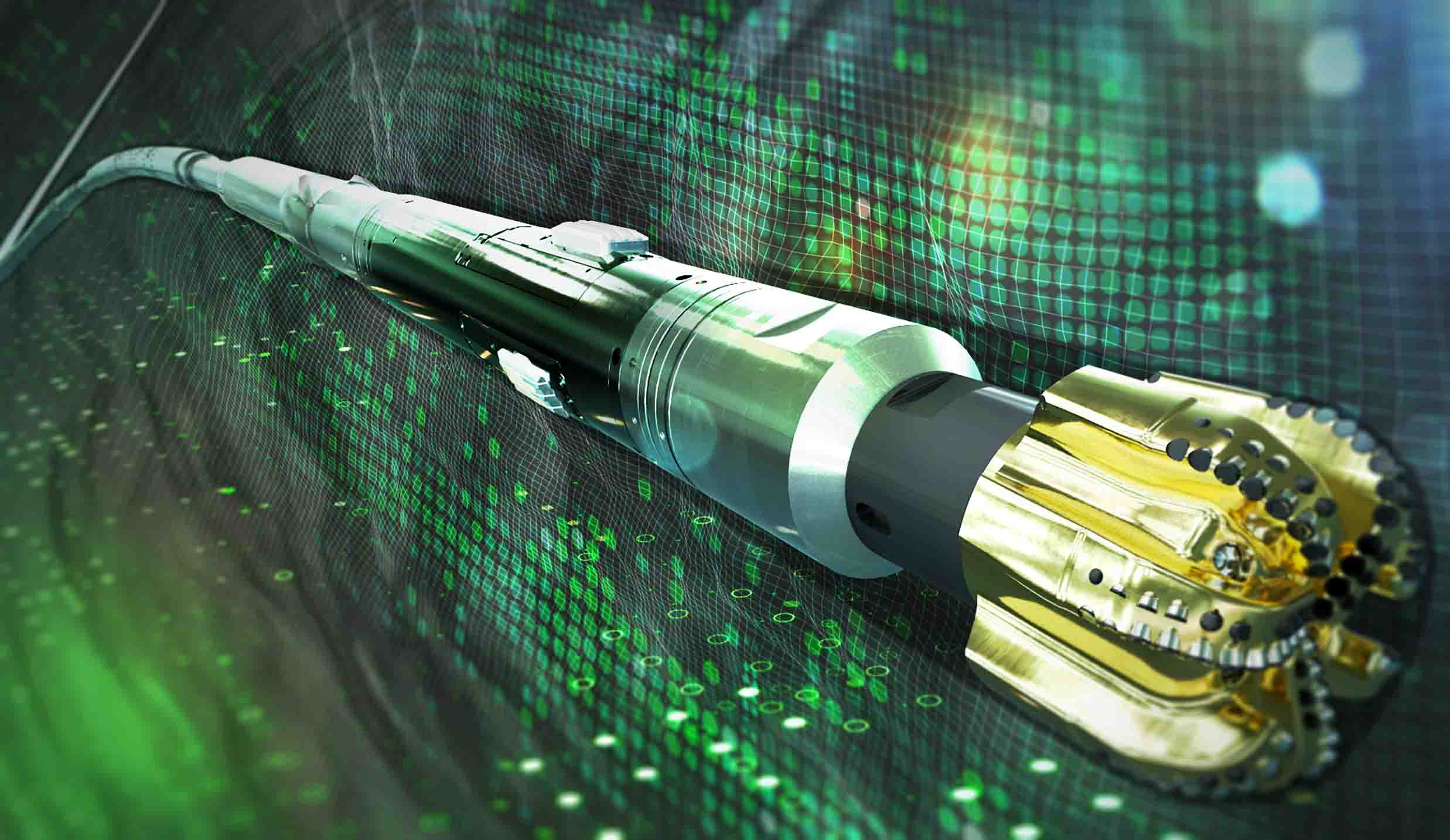
Overcoming obstacles
Repetitive tasks that follow a set of pre-defined rules and instructions are best suited to automation, which is why refineries are increasingly implementing automated processes. From transporting materials and products within the facility to emissions monitoring to process control, automation is delivering efficiencies that lead to enhanced productivity and improved safety.
Although automation has proven its value in downstream operations, the upstream segment of the oil and gas industry has not yet experienced scaling of this technology.
Hesitation is due as much to misgivings about the applicability of the technology in complex and varied operating environments as it is about safety. In an industry characterized by complex processes in harsh operating conditions, assuring safety is critical. The hesitation to automate is often driven by the ambiguity of the input data. In subsurface applications, the data that would be used to automate in a control loop could be uncertain or incomplete.
The biggest barrier to adoption, however, is overcoming doubts that automation can deliver enough value that it is worth changing the way work is done today.
Fortunately, as more functions are successfully automated and positive outcomes demonstrate reliability, trust is building among stakeholders, and automation is gradually proliferating.
Discrete processes are being automated in nearly every type of energy industry activity, and these successes prove that automation has the potential to deliver value in every step of the field development life cycle.
Powering insights
Intelligent tools provide data-driven insights that enable better decision-making in well planning operations.
For some organizations, implementing a digital twin is a fairly new undertaking, but for Baker Hughes, it is already an integral part of developing a drilling plan. Well designs are improved through extensive iterative modeling with a digital twin that uses the best available data to produce an optimal well plan.
To ensure a well is drilled within planned parameters, the digital twin runs simulations for the entire drilling program, taking into account formation properties, temperatures, pressures, flow rates, wellbore trajectory, casing and completion design to identify the best possible scenario. This reduces the likelihood of encountering unexpected issues that could compromise a successful project and allows the plan to be continually optimized for efficiency.
Digital twins are also leveraged during real-time operations. Instead of the model being fed with simulated data, real-time data allows the model to continuously update, resulting in a system that is geared toward finding the optimum setpoints for well construction, which can be used as inputs for autonomous operations.
Achieving autonomous operations requires a digital well construction platform that enables holistic operations management. This is where a platform like Corva’s—a Houston-based company that delivers cloud-based well construction digital solutions—comes into play.
In addition to enabling visualization at the rig, asset or fleet level, Corva’s digital platform includes more than 100 applications that allow process optimization throughout the well construction process.
Moving from data to drilling
Data is foundational to performance improvement, but collecting accurate data is just the first step on the road to automation. Data is used in three primary ways that enable increasingly sophisticated operational control.
The first is descriptive, which in simple terms is collecting and reporting performance data. Descriptive data is static, and experts must evaluate it and determine if action should be taken, for example, to adjust the power level on a piece of equipment or change the frequency with which a task is executed. If there is no human intervention, nothing changes operationally.
Predictive models apply descriptive information to provide a forward look at operations based on historic and real-time performance data. In this case, the system not only examines current conditions but also forecasts future ones such as when a piece of equipment will need to be replaced.
Prescriptive modeling uses historical and performance data to assess, analyze and take action independently. For example, the system can change the choke on an engine or limit the number of times a valve opens and closes to reduce wear. In this instance, the system detects an issue, performs an analysis, determines the best action to take and then executes that action to alter the outcome.
Before the industry is ready to accept the outputs of a prescriptive model and trust in its ability to automate operations, there must be evidence that proves descriptive and predictive automations are possible.
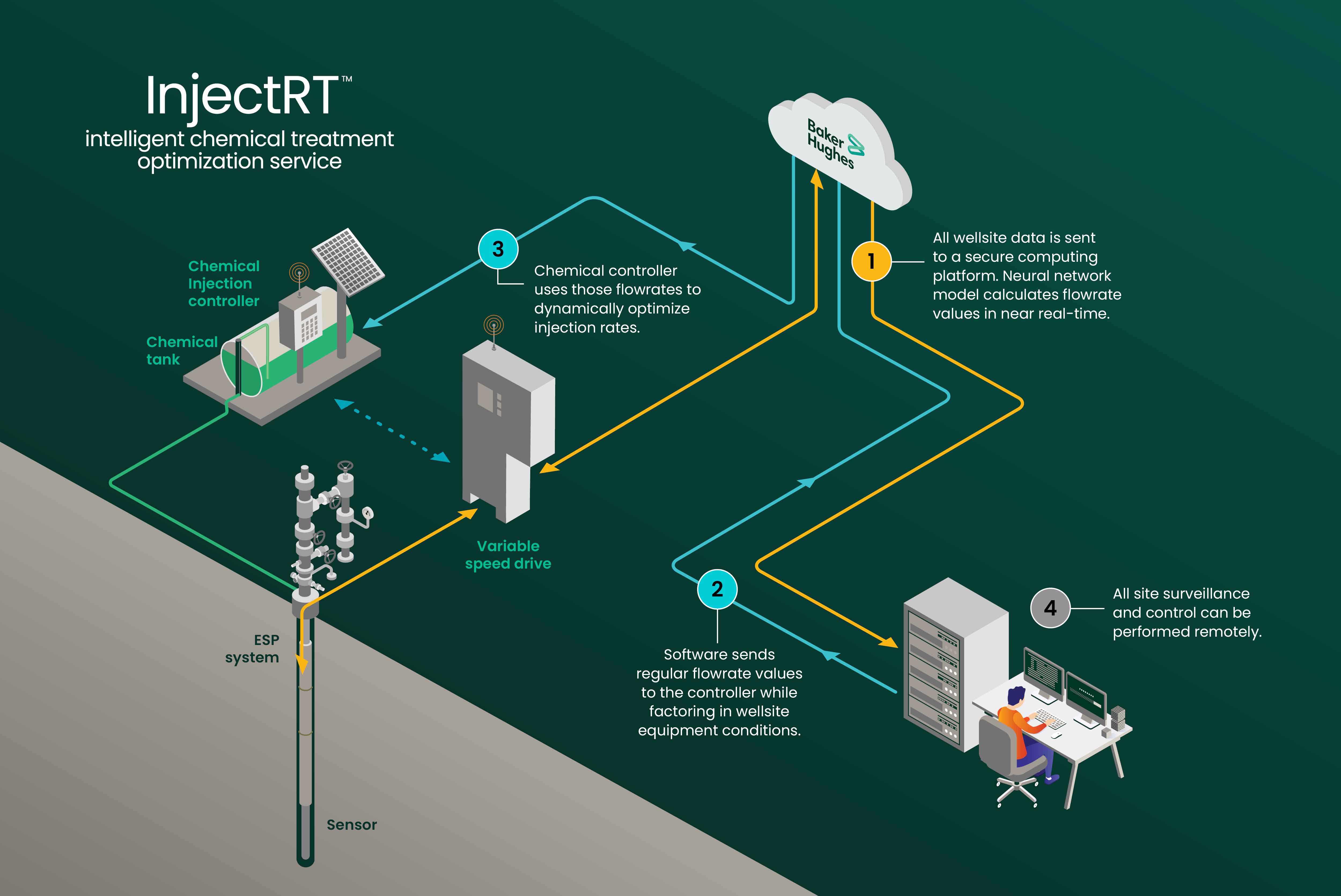
Earning trust
Based on more than 100 years of drilling experience, the Baker Hughes i-Trak drilling automation service demonstrates real-time wellbore placement can be automated to improve accuracy and efficiency.
The system is a paired hardware-software service that allows the execution of automated well placement. Data from downhole tools, rigs and third-party sensors are sent to the edge automation server, which hosts time-critical applications that cannot be executed in the cloud. The server executes microservices that automate the steps of the well construction process, sending commands to the rig control system to enable full closed-loop automation.
The server is the mastermind of automated operations and coordinates decentralized functions managed from the cloud as well as functions executed directly within downhole electronics, such as automated steering. Through the Baker Hughes IoT platform the system is connected bidirectionally to a remote operations center that is staffed by a team of experts for 24/7 oversight.
The i-Trak system can be deployed in multiple modes that allow operators to approach projects with different levels of automation. The first level is “shadow mode,” in which the system creates a well trajectory based on real-time data and proprietary algorithms. The crew assesses each suggestion before applying those that are deemed appropriate using traditional geosteering technology.
The next level of application, “advisory mode,” permits the automated solution to be performed by i-Trak’s reservoir navigation system (RNS) once the driller approves the action. At this stage, the driller is overseeing operations but not manually intervening.
The most advanced level is full automation, in which the RNS detects and analyzes downhole conditions and physically changes the well trajectory for optimal penetration in the best part of the reservoir.
This capability was put to the test by Equinor in Norway’s offshore Johan Sverdrup field. Using deep-reading resistivity inversions, i-Trak generated navigation proposals that were translated into steering commands and automatically transmitted to the bottomhole assembly for implementation downhole. This enabled precise placement of a high-quality wellbore an average of 1 m from the reservoir roof with minimal dogleg severity (1.3-degree/30 m average). Approximately 70% of the reservoir footage was drilled with automated directional drilling technology, resulting in a smooth completion run drilled 17% under budget.
Automating optimization
While the primary goal of the RNS is to keep the well in the most productive part of the reservoir, real-time drilling data also can be used to make other decisions to improve operations. For example, it is possible to autonomously monitor drilling fluid and predict the fluid composition required for the next operational step to achieve the best outcomes.
Drilling data also can be used for drill bit optimization. Analysis of downhole and operational data indicates how effectively the bit is penetrating the formation and provides insights that allow suboptimal performance to be identified. This information can be used to determine how changes in the bit design could improve ROP. With drilling performance data in hand, it is possible to quickly customize cutter placement on the drill bit for a specific application. In short, better data enables better bits.
Automating production
In an industry increasingly focused on efficiency, it is important to reflect that the shortest cycle barrel is not delivered by drilling new wells; it is delivered by optimizing producing wells that are underperforming. By that logic, production automation should be ahead of drilling automation, but the reality is that it lags behind.
Production is a complex conglomerate of equipment, operational and reservoir variables that continuously change over a longer period of time. Each field also presents a different set of challenges. From well-specific parameters, such as an optimized chemical treatment and carefully selected downhole equipment, to field-level constraints, such as fluid-handling capacity and power quality, each project has a unique combination of variables that interplays in the production process.
Most ambiguous is the movement of liquids and gas between the wells, where it cannot be observed or measured. Field or reservoir drainage is ultimately about the recovery of hydrocarbon liquids or gases present in the field through the mechanism of the wells.
In addition, there is significantly less capital investment from operators in this segment. While the value is high, the execution is complex. Most investments are opex, which factor directly into the cost per barrel of production.
The goal is to minimize this expense while optimizing economic recovery. For fields or wells producing over decades without a concerted effort over time to collect and preserve this measured data, less can be inferred about what factors impacted historical production.
To effectively orchestrate these disparate components in a cohesive way, there must be seamless coordination across the entire system. The solution must account for the distinctive characteristics of each field and provide a scalable way to deploy automation.
In recent years, companies have been preparing for this future by focusing on data management, aggregation and visualization. Moving forward, they are building on that foundation to gain efficiencies through increased automation.
Implementing production technology requires a cohesive orchestration layer—a single pane of glass experience to manage the complexity of the field. This experience is supported by:
- Advanced analytics capabilities to deliver insights;
- The ability to easily integrate core operating data, often located in disparate systems;
- Secure connectivity to real-time operating data, required for everything from alarming to feeding analytics;
- Smart hardware to enable reliable and effective operations; and
- Domain expertise and a solutions-focused approach.
It sounds like a tall order, but solutions deployed today are proving that it is not mission impossible.
Baker Hughes is applying AI to deliver advanced analytics for electric submersible pumps (ESPs) using its proprietary Leucipa production automation technology. The analytics applied to ESP performance leverages sophisticated ML models to detect potential failure conditions, such as sanding or scale. Building the ML model began with gathering historical data for training. In this case, the company’s 24/7 artificial lift monitoring team supported the training process by providing specialized knowledge of critical condition trends.
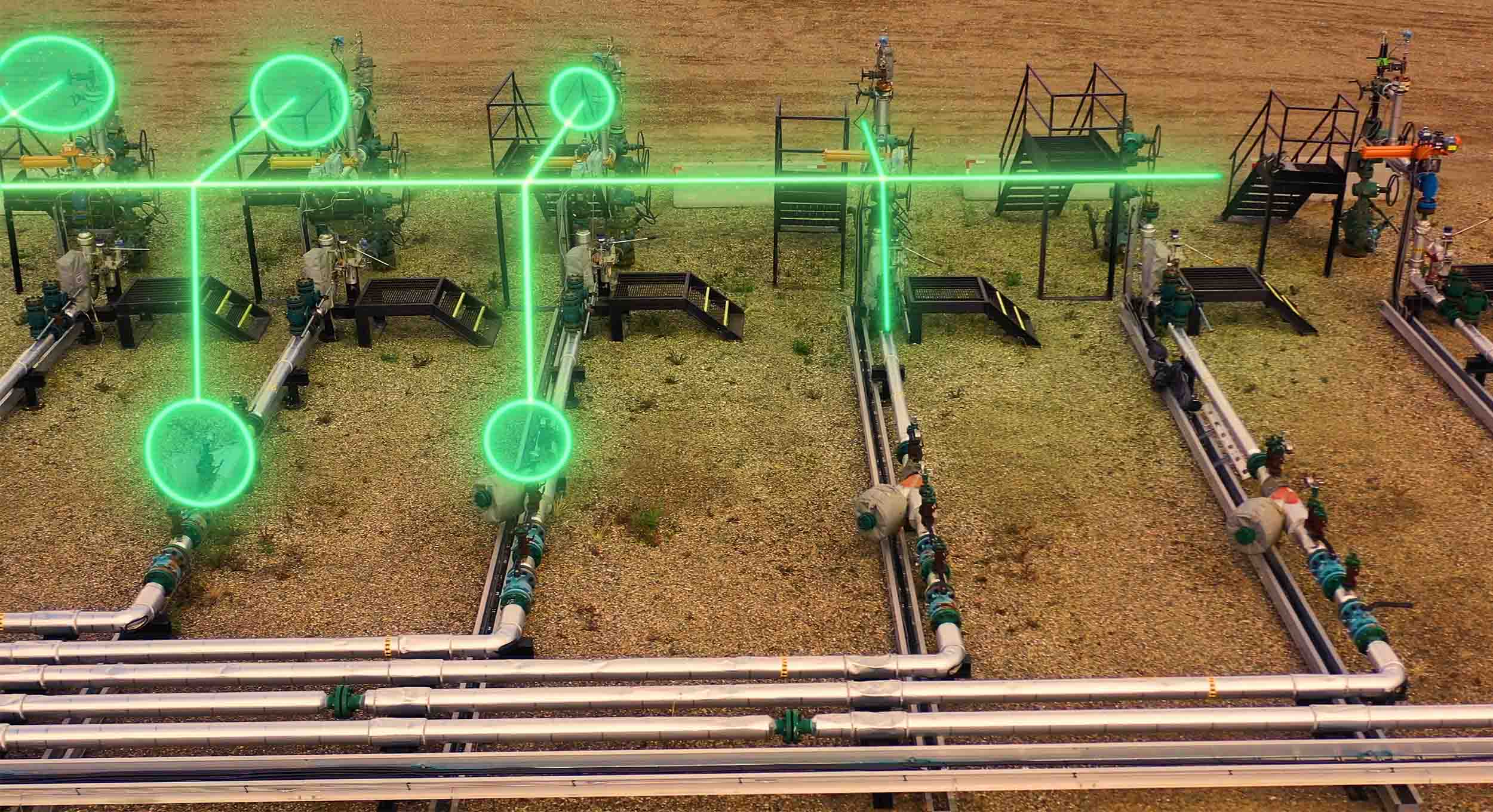
Leucipa’s ESP AI software analyzes data from the equipment, identifies indicators that failures are likely to occur and provides warnings so adjustments can be made to the pumps.
In addition, an ensemble model integrates multiple failure conditions into a predictive framework that issues alerts and suggests steps that can be taken to prevent failures. This solution has accurately detected more than 3,000 critical conditions in one operator’s field in the span of 30 days.
But the intent of this technology is not to provide failure prediction but to provide optimization recommendations utilizing AI-based failure prediction coupled with physics-based modeling.
Physics models are important as both standalone engines and as a companion to ML. For example, physics models can create synthetic data for ML models when actual data is missing.
One example of analytics-driven, closed-loop automation is the recent deployment of a solution designed to autonomously optimize chemical dosing based on real-time insights gathered from an ESP.
Control and telemetry data are sent to the cloud, where a physics-based model leverages a neural network to calculate key parameters like flow rates. Those values are sent to a chemical injection controller that uses flow volume to calibrate the amount of chemical to dispense downhole and then ensures that the precise volume is injected. This process demonstrates how analytics can be connected to smart hardware to drive closed-loop automation.
Applying this solution inhibited scale and optimized chemical treatments in real time. The operator monitored field operations to capture metrics demonstrating that automation decreased power consumption and reduced emissions. Over a 90-day period, this solution delivered 29,000 incremental barrels of production per day across the customer’s wells.
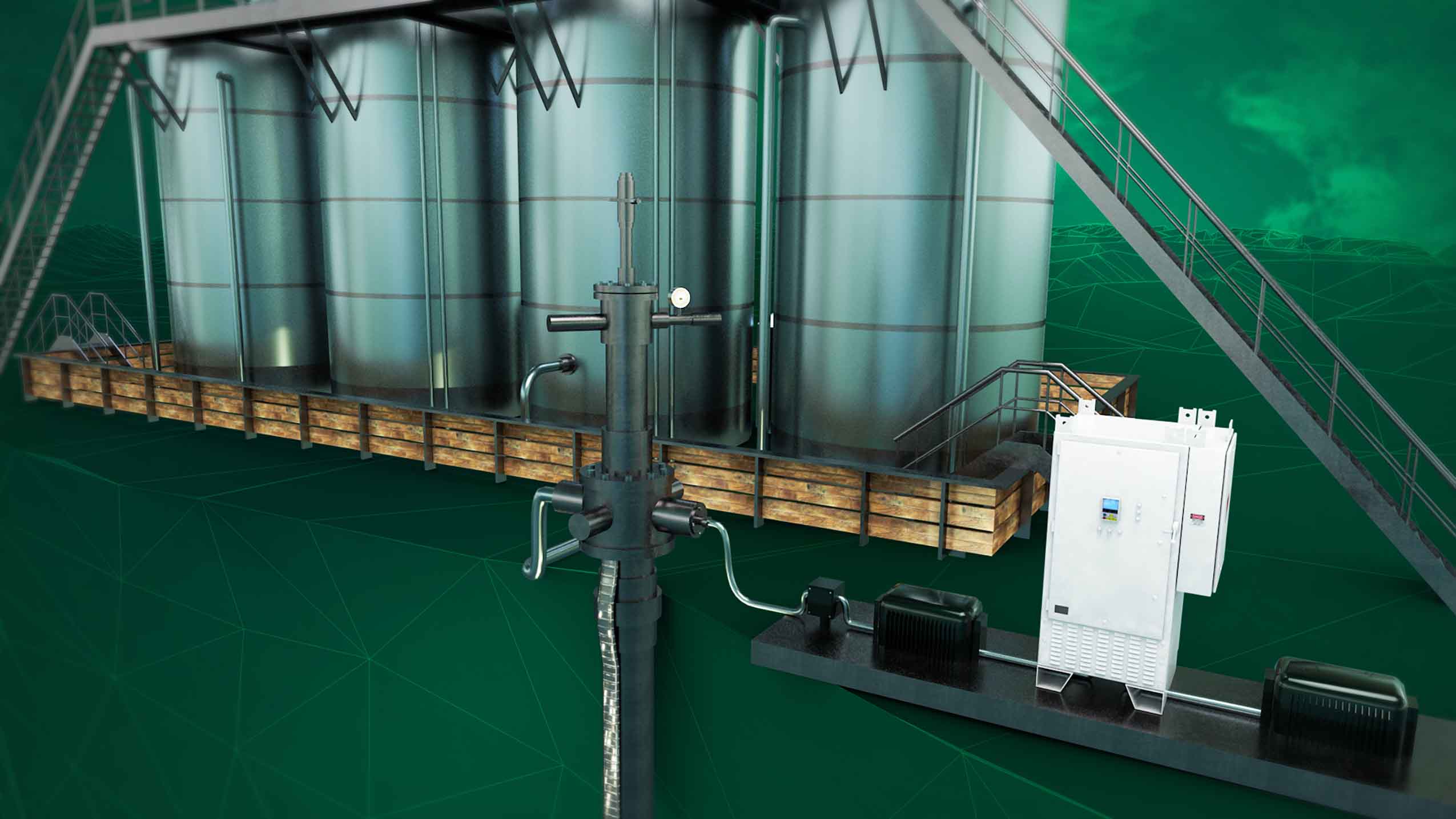
On the horizon
Oilfield automation is continuously evolving, and existing solutions clearly show that automation is becoming an increasingly integral part of reservoir assessment, well planning, drilling and production.
Applied automation is already delivering gains in productivity. Strong results to date, coupled with the continuing pressure to get more value from existing assets, will be a strong driver for expanding and extending this technology. Ongoing investment in R&D will fund a pipeline for developing next-generation algorithms, methodologies and technologies that will improve today’s automated processes and facilitate even more automated functions.
The advent of generative AI will allow access to more information, further improving the certainty required to automate. At some level, generative AI pulls together validated data from many sources, especially previously unstructured reports and supporting data, to improve confidence. Advances in artificial reasoning will allow simple, rule-based systems to become more powerful at scale. These advances in reasoning also will deal with much more complex scenarios that humans deal with today.
Improvement in automation requires trust; however, the uncertainty in which the E&P sector operates can slow down the process of building that trust. This can be accelerated by leveraging change management practices as a part of adopting new solutions.
API is already planning for this future, forming committees to address the challenges ahead and charging them with developing a pathway for standardization. Intelligent systems could soon be connected to every rig and every well, managed through a single interface to achieve even greater efficiencies and increased production while improving worker safety and enhancing environmental stewardship.
A future characterized by highly autonomous operations and end-to-end improvement is not far beyond the horizon.
Recommended Reading
Shale Outlook Permian: The Once and Future King Keeps Delivering
2025-01-11 - The Permian Basin’s core is in full-scale manufacturing mode, with smaller intrepid operators pushing the basin’s boundaries further and deeper.
Permian to Drive Output Growth as Other Basins Flatten, Decline–EIA
2025-01-14 - Lower 48 oil production from outside the Permian Basin—namely, the Bakken and Eagle Ford shales—is expected to flatten and decline in coming years, per new EIA forecasts.
Shale Outlook Eagle Ford: Sustaining the Long Plateau in South Texas
2025-01-08 - The Eagle Ford lacks the growth profile of the Permian Basin, but thoughtful M&A and refrac projects are extending operator inventories.
Shale Outlook Uinta: Horizontal Boom to Continue in 2025
2025-01-11 - After two large-scale transactions by SM Energy and Ovintiv, the Uinta Basin is ready for development—and stacked pay exploration.
Exxon: Longer Laterals, Cube Well Design Lowering Permian Costs
2024-12-11 - Exxon Mobil is boosting spending to grow global oil and gas production by 18% by 2030. U.S. rival Chevron Corp. recently said it’s cutting spending in favor of free cash flow.
Comments
Add new comment
This conversation is moderated according to Hart Energy community rules. Please read the rules before joining the discussion. If you’re experiencing any technical problems, please contact our customer care team.